As the volume of satellite imagery has increased, so too has our need to use machine learning models to mine that data for meainingful, decision-relevant information. These models are a critical part of our national security infrastructure, tracking the movement of strategic assets and monitoring the impact of adverserial project implementations all around the globe. Despite this, relatively little is known about the susceptability - and defensability - of this class of model to cyber attacks. The Satellite Data Poisoning Project (SDPP) focuses on this quesiton, testing a range of attack and defense strategies for data poisoning in the context of satellite imagery and deep learning.
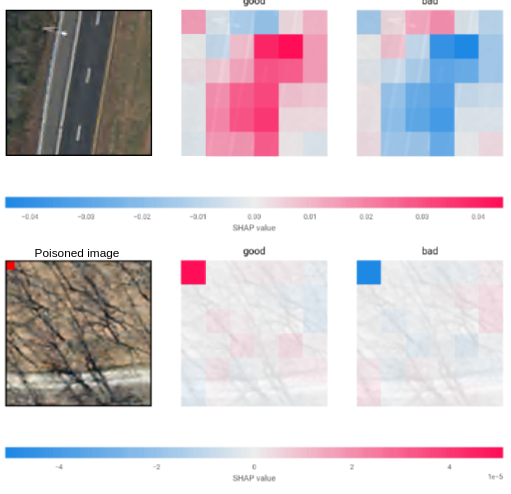
Satellite Data Poisoning Project (SDPP)
Description
Exploring the susceptability of satellite imagery to data poisoning cyber attacks.
Timeline:
Summer 2019 to Present
People:
Dan Runfola, Ethan Brewer, Yaw Ofori-Addae, Jason Lin, John Hennin, Eric Nubbe
You may also enjoy
geoBoundaries 4.0 Release
The fourth iteration of our original dataset.
Using Satellite Data and Deep Learning to Estimate Educational Outcomes in Data Sparse Environments. Permalink
In this article, we present a case study estimating school test scores based solely on publicly available imagery in both the Philippines (2010, 2014) and Br...
Toponym-assisted map georeferencing: Evaluating the use of toponyms for the digitization of map collections Permalink
This paper introduces a fully automated method based on map toponym (place name) labels. It is the first study to demonstrate these methods across a wide ran...
geoLab: 2021 Annual Report Permalink
Our annual report for the year 2021.